Salary survey data is an essential ingredient to any compensation program, but like any tool, it can cause as much harm as good if the wrong tool is procured, or if used the wrong way.
This may seem extreme, but compensation survey data can single-handedly tank a compensation program — and your company. This happens when you use a data source or salary benchmarking tool without understanding how the underlying data is aggregated and consolidated, and therefore the numbers you see are inaccurate. When this happens, the best case scenario is that your executives and employees lose trust in your pay program; the worst case is you overspend and burn through your company resources faster.
Compensation market data comes in all shapes, sizes, and price ranges. From free crowd-sourced resources such as Glassdoor, Payscale, and Pave, to paid company-sponsored surveys such as Radford, Mercer, Willis Towers Watson, and more, it can be hard to know what is right for you.
Below, we will provide you with a set of questions to ask each salary survey provider, along with an in-depth explanation of why!
1. How do you aggregate your data for different roles?
If a company is aggregating roles based on department versus role, this can really skew your results. For instance, in engineering departments, you may have software engineers and machine learning engineers. These are two roles that can have vastly different pay – so if these are aggregated together, you can end up paying more than necessary for talent.
2. How many companies of similar stage and size to my company are in your survey?
Some survey companies will mix all their data together, so it’s important to know what percentage of their participants fit your demographics. You don’t want to be in a survey that consists of only the top-paying tech companies if you are an early retail startup. In this world, the weight of the large companies would skew the median pay or 50th percentile data, and in all likelihood cause you to drastically overpay.
3. How many data points are greater than 12 months old in your salary survey?
Salary surveys have a give-to-get model in most cases. However not every company will participate each year. And sometimes, depending on the company size, this can really skew the report’s numbers. To offset this, surveys will sometimes include “about to expire” data points and apply an “aging” calculation to it. When they do this though, it’s based on assumptions of inflation or what other similar roles saw happen — it’s not actually what the roles are paid. If they do this to a role that actually had a flat year for pay, it can again increase the risk of overpaying.
Ideally, a good survey company is fully purging the data and updating their survey once per year. So no data should be older than 12 months.
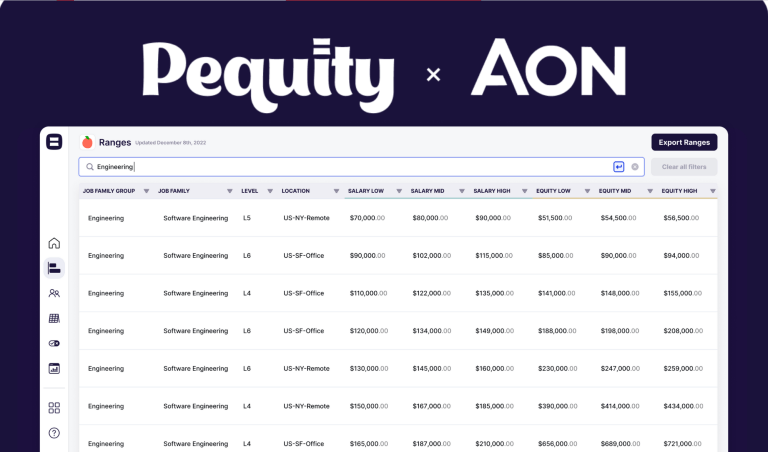
4. Can I filter the compensation data to precisely my stage and size?
This is again an indicator of the maturity and stability of the data from the company. If they don’t provide narrow cuts of their salary data, they are likely mixing multiple stages together to achieve a minimum data threshold. This means you can’t filter the data down, because, without the other supplemental company data they are plugging into the numbers, there would be nothing to show.
It also is a good way to gut-check if there is actually a good sample size for your type of company. For instance, they could say that they have 20 companies of your stage and size, but when asked to filter, you may find that those companies only submitted a small portion of the data. Or that they are in a different industry or their data is too old to use.
5. How do you determine and aggregate job levels?
Levels are one of the most important parts of wages. A senior software engineer is paid very differently than a principal.
So if your survey aggregates levels data through an algorithm based on:
- Title
- Distance from CEO
- Number of employees reporting to them
… this means the data is likely mixing multiple levels together. HRIS data and titles are inherently messy, especially at small companies. You could have a software engineer reporting directly to the CEO at one company, a director of software reporting to the CEO in another, and a CTO reporting to the CEO in a third. In this case, the algorithm for distance from the CEO would aggregate all their pay together so you’d likely overpay for your software engineer and underpay your CTO. Even if you incorporate titles, small company titling tends to be very inflated — would you consider the Director of Engineering at a 20-person startup the same as a Director of Engineering at a 200-person company?
Knowing how the company aggregates and validates their levels could be what makes or breaks your pay plans.
6. How do you handle salary data outliers? Do you remove or keep them in the survey?
Every company has an outlier. That one employee who doesn’t exactly fit the salary range or pay process. This causes issues internally, but also in surveys.
Most salary surveys are a statistical model. This means they are calculating the bell curve of pay across a set of criteria – role, level, and location to name a few. The issue is if there are many outliers — so someone paid extremely, absurdly high, or low — it can skew the bell curve, especially at the tails.
Most reputable surveys opt to remove extreme outliers, but there are some surveys that do not. This can drive up your pay too high for the upper percentiles rendering them unusable or drop the bottom percentiles too low. This means your data would only be useful right around the median values at best, which can limit your ability to strategically compare and understand what the market is actually doing.
7. How is equity aggregated? Is it total equity granted, equity vested, or some other metric?
Ah, equity. Many surveys such as Radford utilize their own special algorithm to calculate the equity amounts and values (Radford uses Black-Scholes Method for reference). Some surveys though do a simple roll-up of equity. This may not seem like a big deal, but the difference in their methodology can be what causes you to burn out of your equity pools quickly.
For example, if a survey provides total equity granted data, you need to account for the fact this data will include new hire grants, refreshes, and promotions — and you may not know how much is actually vesting each year. This means you are likely going to overspend on equity if you use the whole value.
Similarly, if a company is just providing annual equity, this can be useful for making grants, but for leaders and senior roles, it can keep you from competitively paying as many executives will anchor on their total percentage of the company.
All of these factors just get worse too if the survey is not accurately aggregating data by levels, company stage, and role. For example, imagine combining the equity of a Series A CTO with the equity of a Post-IPO company’s CTO. There would be no accuracy.
In either case, you need to understand how and why the equity is aggregated.
8. How many job levels and roles does their compensation survey cover?
Another factor to consider is if the survey contains the roles and levels you care about. Some compensation surveys focus on retail; others on tech. Some have better executive coverage than others.
Know what they have and how much data they have for each or you could end up sharing your sensitive company data to get nothing in return.
A good way to test this is to ask for a list of their levels and roles without the data included.
9. How many locations does the salary survey cover?
Location-based pay is often part of a well-rounded compensation program. If you are planning to staff employees in more than one location, you should double-check if that location is covered by your survey company.
Again, ask if you can have a list of the locations where they have statistically significant data access. Don’t just ask for a general list; the data means nothing if it’s based on one-employee data point.
10. Can you get a list of the locations, roles, job levels, and participating companies?
Most good salary surveys will provide you with a list of all their roles, levels, locations, and participating companies. They should be able to tell you the data quantity for each as well. This will help you assess the situation more closely.
Conclusion
Using salary survey data is essential in creating an effective compensation program for any organization. However, it can cause harm if the underlying data is not properly understood or is inaccurate. To avoid such situations, a healthy salary survey or compensation data set should provide accurate and up-to-date data tailored to the organization’s specific size, stage, and industry. The questions listed in this article can help determine the quality of salary survey data, such as how the data is aggregated for different roles, the number of companies similar in size and stage to your company, and how the company handles outliers. Knowing the answers to these questions can help ensure that your compensation program is competitive and equitable, leading to employee trust and retention.
We encourage you to copy and paste the list of questions above directly to your survey providers to see what they say!
Obviously from the above, it can seem overwhelming to choose a good data source — if you want help, Pequity can refer you to our data providers, and based on your preferences we’ll advise you on which ones would be the best fit.